Two Fundamental Types of Study Questions
Specifying the research questions is essential to selection of an appropriate study population. There are two fundamental types of research questions that have important implications for selecting an appropriate study design.
Descriptive Research
Descriptive research aims to accurately estimate and describe the frequency of health outcomes and health-related exposures in the population; this requires a representative sample.
- Did death rates for heart disease vary by state in 2011?
- What proportion of high school students smoke? Or use drugs?
- What is the frequency of death from coronary artery disease among black and white males and females, and how have those rates changed over the past 20 years?
- What proportion of the adult residents of Weymouth, MA has type 2 diabetes or hypertension? What proportion are obese?
Questions like these require samples that are representative of the population being studied, that is comparable to the population in their characteristics. As with all studies, they also require adequate sample size in order to minimize sampling error and to obtain accurate estimates of population parameters.
Analytic (Causal) Research
This second fundamental type of research, analytic research, aims to identify determinants of disease by comparing groups of people to identify valid associations between exposures and health outcomes. This requires more restricted samples, as for example,
when The Physicians' Health Study recruited over 22,000 male physicians in the United States in 1981 to test the efficacy of low-dose aspirin (versus placebo) in preventing myocardial infarctions (heart attacks). Instead of enrolling subjects representative of the general population, they wanted to enroll a large sample of subjects who would be easy to follow for a long period of time. Physicians in the United States are registered and easy to track down, even if they move. They also wanted to enroll subjects whose age put them at risk for developing a heart attack in order to have a sufficient number of "events" to do an adequate analysis. Therefore, they enrolled subjects who were 40 to 84 years old. They also restricted the study to males, because in 1981 there were relatively few female physicians in this age range. While these restrictions increased the likelihood of achieving a successful study with a valid conclusion, they limited the ability to generalize the findings to the general population since the sample was not representative.
Questions like these also require an adequate sample size to precisely assess the strength of an association, but they differ from questions aimed at estimating frequencies in the overall population in that that they require making comparisons, e.g., comparing risk between exposed and non-exposed persons. When trying to answer questions like these regarding etiology, it is not so important that the samples be representative of the overall population. Instead, the key is to compare groups that are comparable to each other with respect to other factors that affect the outcome (so-called "confounding factors").
In the aspirin study the investigators also allocated subjects to the treatment groups randomly in order to ensure their comparability. Questions arose later regarding the applicability (generalizability) of the results to women and even to males who were not physicians, but at least the investigators could confidently conclude that low-dose aspirin had significantly reduced the incidence of myocardial infarction in the subset of the population they had studied. In fact, the random assignment of over 22,000 subjects achieved remarkable comparability among the comparison groups with respect to many known risk factors for heart disease.
Drawing Samples from a Population
Drawing Representative Samples for Estimating Population Parameters
When the goal is to draw a sample that is representative of the population in order to estimate population parameters, one can simple draw a simple random sample, meaning that selection is done by any method such that each individual in the study population has an equal chance of being selected, and the selection of any member does not influence the chances of any other member being selected.
Ideally, one would identify a sampling frame, i.e., a complete list or enumeration of all of the population elements (e.g., people, houses, phone numbers, etc.). Each of these is assigned a unique identification number, and elements are selected at random to determine the individuals to be included in the sample. As a result, each element has an equal chance of being selected, and the probability of being selected can be easily computed. This sampling strategy is most useful for small populations, because it requires a complete enumeration of the population as a first step.
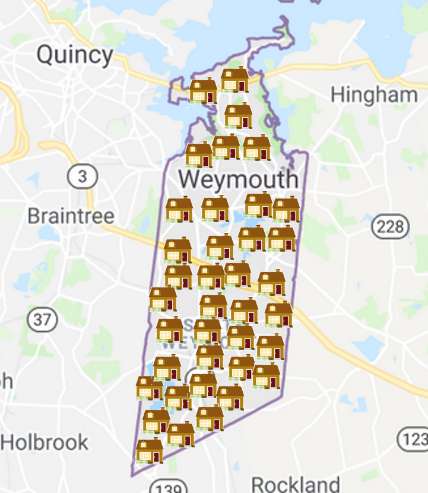
Weymouth, MA conducted a town-wide survey in order to assess the health status of the town. The survey was mailed to a random sample of 5,054 households in Weymouth, stratified by zip code to ensure a representative sample from the entire town. Of these, 3,201 surveys were completed and returned, giving a response rate of 63.3%.
Random Selection
Many introductory statistical textbooks contain tables of random numbers that can be used to ensure random selection, and statistical computing packages can be used to generate random numbers. Excel, for example, has a built-in function that can be used to generate random numbers, and statistical packages such as R can also generate random numbers.
Drawing Samples to Identify the Determinants of Health and Disease
Ultimately, we would like to identify the causes of health and disease, but establishing causal relationships requires that a number of conditions are met, and we will explore this in more detail in the next section of this week's materials. For now we can simply ask "Does a certain exposure (E) cause a particular health outcome (O)?"
Exposure (E) Outcome (O)
The primary goal of analytic research is to identify determinants of health and disease. The putative causes are generally referred to as exposures, and the potential results are referred to as health outcomes.
An exposure is any measurable characteristic that differs across individuals and might affect or be associated with health or disease. Potentially relevant exposures may include any of the following:
- Demographics: Age, sex, ethnicity, religion, occupation, socioeconomic status (SES)
- Behaviors: Smoking status, level of physical activity, diet
- Environment: Pesticide levels, air pollution exposure, heavy metals
- Policies and Laws: Gun laws, cigarette taxes, trans fat bans
- Health states: Diabetes, high blood pressure, depression
A health outcome is any measurable disease, disability, injury, infection, syndrome, symptom, biological or subclinical marker, or health state (positive or negative). Examples might include:
- Death
- Development of a cancer
- Development of type 2 diabetes
- Pregnancy
- Development of hypertension (high blood pressure)
- Becoming infected with tuberculosis or developing any other infection
- Having a positive skin test for tuberculosis
- Does hypertension increase the risk of stroke?
- Does obesity increase the risk of hypertension?
- Does obesity increase the risk of type 2 diabetes?
- Does having type 2 diabetes increase the risk of coronary heart disease?
- Medical records and reports of diagnostic tests
- Health insurance data bases
- Surveillance data from local, state, or federal health data bases
- Disease registries
- Death certificates
- Self-reports
Note that conditions like diabetes and hypertension can be regarded as either an exposure or a health outcome, depending on the question that is being asked, as illustrated in the examples below.
For the first question hypertension is the exposure of interest, and stroke is the outcome of interest. For the second question obesity is the exposure, and hypertension is the outcome of interest.
Another example:
For the first question type 2 diabetes is the outcome of interest, but for the second question it is the exposure of interest.
Methods of collecting data on health outcomes are varied and might include: